Guido Conaldi and Francesco Mambrini
What’s happening with AI in Higher Education?
It’s an exciting time to be in Higher Education (HE). The landscape is transforming, and at the centre of it all, there’s a tech phenomenon that’s hard to ignore: AI-powered chatbots, like ChatGPT. Research in this area is exploding, spanning multiple facets of AI in HE (e.g., Perkins 2023; Kasneci et al. 2023; Fauzi et al. 2023; Mollick and Mollick 2022; Mollick and Mollick 2023). While we sense an overall positive sentiment towards this new tech, concerns around its impact on academic integrity are also surfacing.
So, what’s the problem?
As researchers, these concerns resonate with us. We’ve had similar conversations with lecturers and know that they share these worries. The anxiety isn’t unfounded – the potential for students to use tools like ChatGPT in ways that don’t promote actual learning is real. That’s why we believe the key remains a shift towards more authentic assessment paired with the integration of AI-powered tools in our curricula. Authentic assessments, by design, encourage original thinking and the application of knowledge in real-world contexts, making it less likely for students to use AI-tools inappropriately – especially when their appropriate uses become part of the learning process.
What’s being done about it?
Some of the recent literature provides recommendations for identifying the inappropriate use of AI. Some researchers we’re following offers suggestions for detecting possible unfair use of tools like ChatGPT in academia (e.g., Cotton et al. 2023; Gimpel et al. 2023; Rudolph et al. 2023). Two such recommendations stand out.
The first one suggests using AI-powered detection tools. This sounds promising, but there are caveats. These tools are expensive and have been adopted by only a few universities so far because their reliability is yet to be fully tested. Plus, this approach likely leads us into a cat-and-mouse game with ChatGPT and similar AI tools, where each continually adapts to outsmart the other.
The second strategy focuses on examining certain linguistic features. This comes down to two intertwined aspects: identifying linguistic telltale signs of a text being ChatGPT-generated, and the idea – simply put – that you need to know your students and their writing style.
The first component has to do with linguistic cues that supposedly give away an AI-written text. These might be particular sentence structures or use of vocabulary. The second component links to the personal rapport and understanding we develop with our students. It’s an interesting point that emerged during various meetings and workshops on AI in HE. Educators shared their belief that after engaging with a student’s emails, assignments, or CVs, they could ‘tell’ if something was written by ChatGPT. But how reliable is this gut instinct?
These are the suggestions that have captured our curiosity. Do they stand up to scrutiny? Not just in the long run, but right now? That’s the question we’re setting out to answer. Of course, we know that right now (how long for?) ChatGPT is prone to make up academic references and copy-pasted reference lists can be easily spotted. What about texts where references are not needed though, or texts where blatantly fake references have been removed?
What are we doing about it?
For our initial investigation, we opted for an essay assigned to undergraduate business school students during the 2020-21 academic year. We collected the over 100 essays that the students had submitted at the time. But we needed the AI’s take on the same assignment too.
So we prompted ChatGPT (version 4.0) multiple times and in several different ways to produce essays for the same assignments. We were careful to mimic the conditions the students had when they wrote their essays. Initially, our prompts to ChatGPT followed the original instructions for the essay very closely. But then, we added another layer.
To ensure the bot tried to mimic a student’s writing style, we inserted additional guidelines. For example, we asked ChatGPT to write the essay “in the style of an undergraduate student trying to produce a good essay but with limited academic vocabulary and include a few errors in the construction of sentences.” For each prompt, we produced 20 AI-written essays.
So, now we had a mix of human and AI-written essays, ready to put the detection suggestions to the test. Can we really tell the difference?
Now what?
To explore the language of the essays written by ChatGPT and compare it to those submitted by the students, we used software for automatic linguistic analysis. Such tools are capable, for instance, to tell which word in a sentence is an adjective and which a noun, or to find the direct object of a verb. The software that we used is called UDPipe.
Our study is ongoing, but our early findings are already fascinating. Which features of style would any of us immediately associate with the writing of a bot? Would you say that the style of a machine is more consistent or erratic? more flourished or parsimonious? From our exploration of more than a hundred between different classes of words (nouns, adjective, prepositions, etc.) and their syntactic relations (direct or indirect objects, subjects etc.), it turns out that ChatGPT is very consistent, much more so than the students! And what’s more, its use of those categories of words is in line what students do on average. So, for instance, ChatGPT uses close to the same number of articles per sentence than students, consistently.
What about punctuation? Even there, the essays authored by ChatGPT are more consistent and generally in line with the average of the students’ essays. But what is more interesting here is the fact that, in the essays written by the students the use of punctuation increases with the vote band: texts that were graded higher use noticeably more punctuation marks. And ChatGPT-generated essays (both prompts) behave like the top-graded essays (see Figure 1).
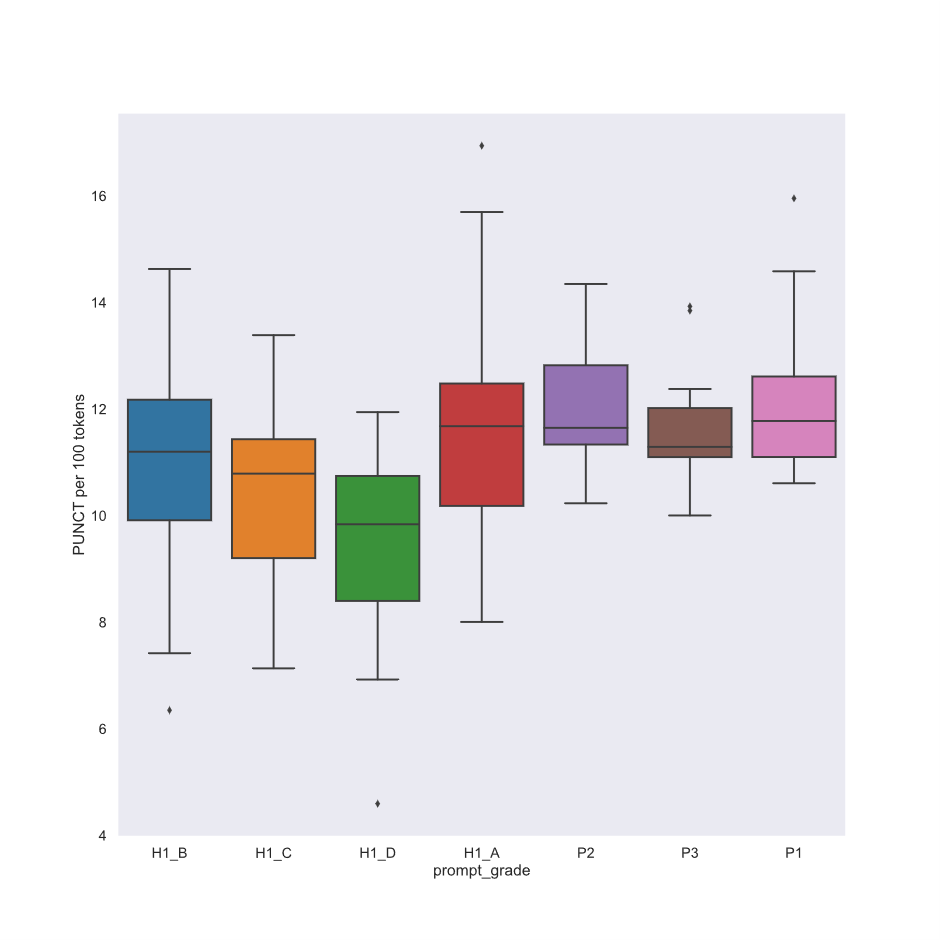
Figure 1 Boxplots of the distribution (x 100 tokens) of punctuation marks, and lexical density for the student-authored vs GPT-generated texts. P1-3 are ChatGPT-generated essays grouped by prompt. In red are essays of students graded in the 70+ band.
But what are the hallmarks of ChatGPT’s style, after all? ChatGPT does seem to have a few preferences – currently, at least: it uses longer words and, in particular, more adjectives per sentence than students. Would we associate those traits with ChatGPT, without knowing it in advance? When we say “after a while, one can tell” are we looking at the right cues – albeit subject to change as the technology evolves – or are we bound to let our own bias seep through and influence our judgement.
and so what?
The advent of AI in HE isn’t necessarily a threat to academic integrity and empirical investigations like ours seem to warn us from approaching it in those terms; rather, it pushes us even more to reimagine our assessment methods and strive for authentic learning. Stay tuned for more findings from our investigation and join the conversation on the future of AI in HE.
References
Cotton, D. R., Cotton, P. A., & Shipway, J. R. (2023). Chatting and Cheating: Ensuring Academic Integrity in the Era of ChatGPT. Innovations in Education and Teaching International. https://doi.org/10.1080/14703297.2023.2190148
Fauzi, F., Tuhuteru, L., Sampe, F., Ausat, A. M. A., Hatta, H. R. (2023). Analysing the Role of ChatGPT in Improving Student Productivity in Higher Education. Journal on Education, 4(5), 14886-14891. https://doi.org/10.31004/joe.v5i4.2563
Gimpel, H., Hall, K., Decker, S., Eymann, T., Lämmermann, L., Mädche, A., Röglinger, R., Ruiner, C., Schoch, M., Schoop, M., Urbach, N., Vandirk, S. (2023). Unlocking the Power of Generative AI Models and Systems such as GPT-4 and ChatGPT for Higher Education: A Guide for Students and Lecturers. http://dx.doi.org/10.13140/RG.2.2.20710.09287/2
Kasneci, E., Seßler, K., Küchemann, S., Bannert, M., Dementieva, D., Fischer, F., Gasser, U., Groh, G., Günnemann, S., Hüllermeier, E., Krusche, S., Kutyniok, G., Michaeli, T., Nerdel, C., Pfeffer, J., Poquet, O., Sailer, M., Schmidt, A., Seidel, T., Stadler, M, Weller, J., Kuhn, J., & Kasneci, G. (2023). ChatGPT for Good? On Opportunities and Challenges of Large Language Models for Education. Learning and Individual Differences, 103, 102274.
Mollick, E. R., & Mollick, L. (2022). New Modes of Learning Enabled by AI Chatbots: Three Methods and Assignments. Available at SSRN: https://ssrn.com/abstract=4300783 or http://dx.doi.org/10.2139/ssrn.4300783
Mollick, E. R., & Mollick, L. (2023). Using AI to Implement Effective Teaching Strategies in Classrooms: Five Strategies, Including Prompts. Available at SSRN: https://ssrn.com/abstract=4391243 or http://dx.doi.org/10.2139/ssrn.4391243
Perkins, M. (2023). Academic Integrity considerations of AI Large Language Models in the Post-pandemic Era: ChatGPT and Beyond. Journal of University Teaching & Learning Practice, 20(2). https://doi.org/10.53761/1.20.02.07
Rudolph, J., Tan, S., & Tan, S. (2023). ChatGPT: Bullshit Spewer or the End of Traditional Assessments in Higher Education? Journal of Applied Learning and Teaching, 6(1). https://doi.org/10.37074/jalt.2023.6.1.9
Guido Conaldi (g.conaldi@greenwich.ac.uk) is Senior Lecturer in Economic Sociology at the School of Business, Operations and Strategy, University of Greenwich. He holds an MSc in Sociology from the London School of Economics and a PhD in Social Sciences from the Sant’Anna School of Advanced Studies in Pisa. His research interests lie in the areas of interpersonal and organizational social networks, as well as the teaching of methods in higher education and the impact of technological innovation in higher education. His current research investigates: the social mechanisms contributing to the endogenous formation of structure and hierarchy in self-managing teams; the impact of AI-driven models on teaching and learning in higher education.
Francesco Mambrini (francesco.mambrini@unicatt.it) is a Researcher at the Università Cattolica del Sacro Cuore, Milan. He is currently working on the ERC-funded project “LiLa – Linking Latin,” where he serves as responsible for the development and implementation of the Linked Data architecture and ontologies for the Knowledge Base. Previously, he worked as a Research Assistant at the Deutsches Archäologisches Institut, Berlin, and at the University of Leipzig. In 2012, he was appointed Joint Fellow of the Center for Hellenic Studies and the Deutsches Archäologisches Institut for the academic year 2012-13. Throughout his career, he has collaborated with renowned projects in the Digital Humanities, including The Perseus Projects, where he held the position of Visiting Scholar in 2009 and 2011. Francesco has also been a dedicated collaborator of the Ancient Greek and Latin Dependency Treebank since its inception in 2009.
Twitter handle: @FrancMabr; LinkedIn